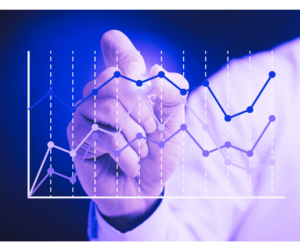
On behalf of Sabina Dobrer
Recently I asked about options for comparing categorical and continuous variables. What to use? Paired? Not paired? So I decided to put this information together to provide some guidelines for the researchers and their teams. In this story I will describe appropriate tests to compare continuous variables.
Paired or Not?
Paired test is used to compare two samples when each individual in one sample also appears in the other sample.
Unpaired test is used to compare two samples when each individual in one sample is independent of every individual in the other sample
Types of tests
There are two types of test widely used: parametric and non- parametric
I personally prefer non-parametric tests for most of the situations, as there is no need to check for the assumptions.
Parametric tests are those that make assumptions about the parameters of the population distribution from which the sample is drawn. This is often the assumption that the population data are normally distributed
Non-parametric tests are “distribution-free” and, as such, can be used for non-normal variables.
Parametric | Non-Parametric |
Paired t-test | Wilcoxon signed rank test |
Unpaired t-test | Mann-Whitney U test or |
Pearson correlation | Spearman correlation |
One way analysis of variance (ANOVA) | Kruskal Wallis Test |
Brief description
Paired t-test: is a method used to test whether the mean differences between pairs of measurements is zero or not.
What you need to test/know in order to use the paired t-test:
- Subjects are independent. For example, you would like to compare student performance on the exam before and after the intervention. In this case we assume that each student does their own work on both exams
- Each of the paired measures are obtained from the same subject
- The distribution of differences in means is normally distributed
- The variance for the pair t-test the variance is not assumed to be equal
Note: the normality assumption is more important for the small sample sizes, as based on the Central Limit Theorem when sample size tends to infinity, the sample means approaches the normal distribution. What is large sample condition? In most of the books 30 is commonly used for the “large enough” sample. As for me, I always check all the assumptions for any test.
Wilcoxon signed rank test: is a non-parametric method used to test whether the median of pairs of measurements is zero or not.
What you need to test/know in order to use Wilcoxon signed rank test:
- Subjects are independent
- Each of the paired measures are obtained from the same subject
- Measures are continuous in theoretical nature
- Ordinal level of measurement to ensure two values can be compared
Unpaired t-test: designed to compare the means of two independent or unrelated samples.
What you need to test for in order to use Unpaired t-test:
- The variance between two groups assumed to be equal
- The data is continuous
- Only two groups are compared
- Groups are independent
- Data should be normally distributed
Mann Whitney U test (Wilcoxon Rank Sum Test): test equality of means of two independent samples or whatever two samples are likely to derive from the same population. You also can interpret the test as comparing medians between two samples.
What you need to test/know in order to use Mann Whitney U test:
- Dependent variable is continuous and ordinal
- Independent variable is categorical with two categories (defines two samples you would like to compare)
- Samples are independent
- In order to interpret the results of this test you need to understand if distribution shape of two samples are the same or different. If the two distributions have a different shape you can only use this test to compare mean ranks
One Way ANOVA: compares the means of two or more independent groups in order to determine whether there is statistical evidence that the associated population means are significantly different. One-Way ANOVA is a parametric test.
What you need to test/know for in order to use One-Way ANOVA
- Normality – that each sample is taken from a normally distributed population
- Sample independence – that each sample has been drawn independently of the other samples
- Variance equality – that the variance of data in the different groups should be the same
- Measures are continuous in theoretical nature
Kruskal Wallis Test (one way analysis of variance) and test whether the samples originate from the same distribution. This is an extension of the Mann Whitney U test where independent variable has more than 2 categories (comparing more than 2 samples). A significant Kruskal Wallis test indicates that at least one sample stochastically dominates the other sample. Note: stochastically means in random. We mostly use it to determine if there are statistical difference between two or more groups of independent variable on a continuous or ordinal dependent variable.
What you need to test/know for in order to use Kruskal Wallis Test
- Dependent variable is continuous and ordinal
- Independent variable is categorical and can include 3 and more categories
- Observations must be independent
- In order to interpret the results of this test you need to understand if distribution of each groups are the same or different. Same as for Mann Whitney U test if distributions have a different shape you can only use this test to compare mean ranks
Pearson correlation: is the most common parametric way of measuring a linear correlation. It is a number between –1 and 1 that measures the strength and direction of the relationship between two variables.
What you need to test/know in order to use Pearson correlation
- Both variables are quantitative
- The variables are normally distributed
- The data have no outliers
- The relationship is linear and relationship between the two variables can be described reasonably well by a straight line
Spearman: non-parametric alternative to Pearson correlation.
What you need to test/know in order to use Spearman correlation:
- The variables are ordinal
- The variables aren’t normally distributed
- The data includes outliers.
- The relationship between the variables is non-linear and monotonic
I hope this helps. You can listen to my previous Lunch & Learn lectures in online format here WHRI Lunch & Learn Series – Women’s Health Research Institute.
Go to our Stats corner in eBlast for previously published tips in data management and analysis eBlast Archive – Women’s Health Research Institute (whri.org).